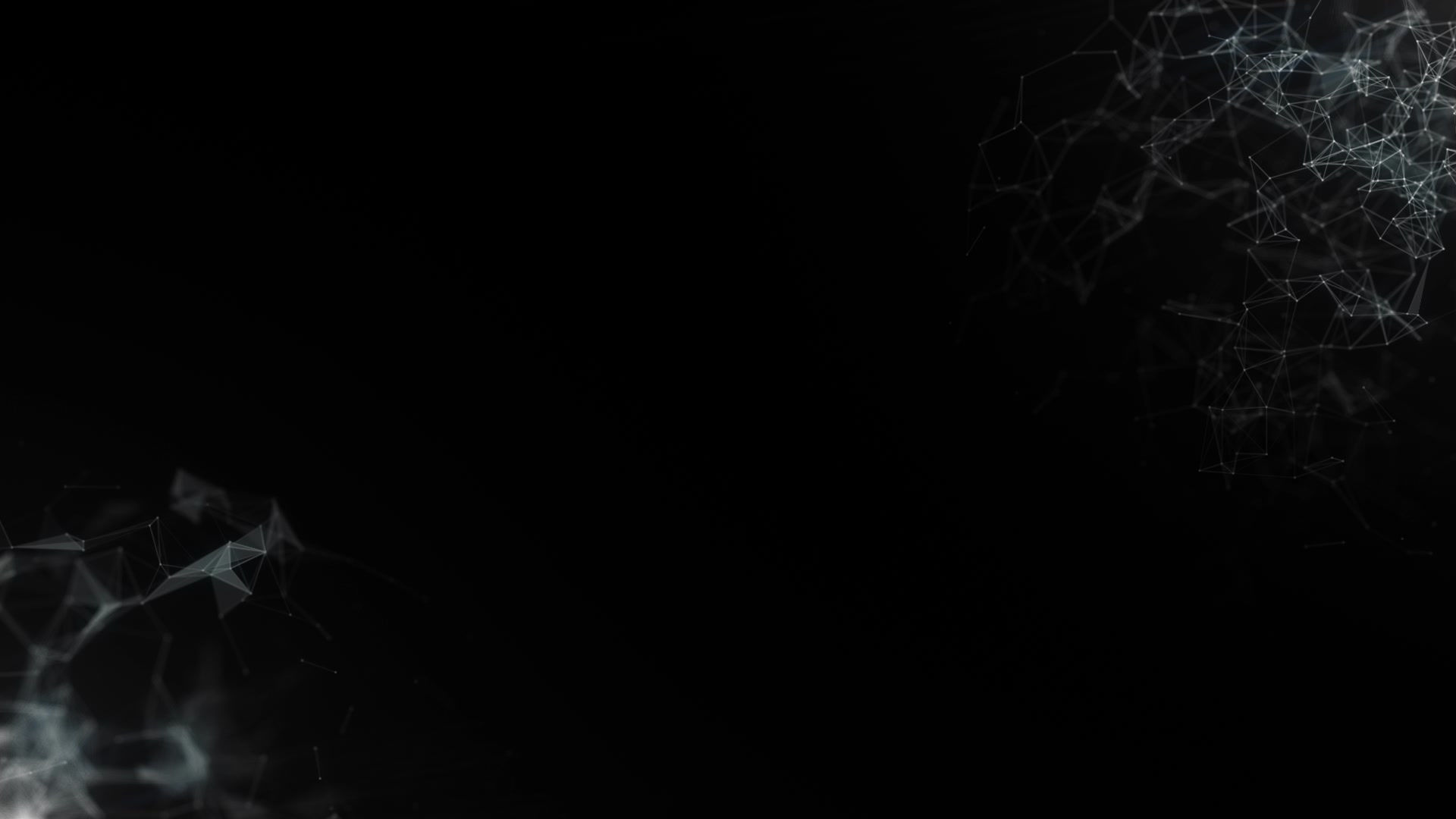
Constraining distributions of galaxy properties in massive datasets:
A Hierarchical Bayesian approach
Cosmological redshift surveys are in a large-N (number of galaxies), low signal-to-noise (S/N) regime. The relatively low quality of the data for individual galaxies significantly hinders any galaxy population analysis performed from an object-by-object approach.
However, we are not really interested in the exact value of any galaxy property (e.g. colour, magnitude, velocity dispertion) for a particular galaxy. What we really need, in order to understand the way a galaxy population evolves, is the distribution of galaxies in physical parameter space. Our goal is therefore to constrain the hyperparameters of a model for the population distribution, marginalizing over the physical parameters of individual galaxies.
Hierarchical Bayesian techniques provide the ideal framework for maximizing the amount of information that we can extract from massive, low-S/N surveys. Let's assume that we have a physical parameter vector a (e.g., intrinsic colour), the distribution of which we want to constrain, and an associated data vector d (e.g., observed colour). The goal is to model the pdf of survey galaxies in physical parameter space as a function of some global hyperparameters (i.e., the width and mean value of a Gussian distribution, in a simple example), that we can constrain.
In Bayesian terms, the idea is to construct the likelihood of a given set of hyperparameters given the data d. From the Bayes' Theorem we know that this likelihood is proportional to the probability of measuring d given a set of hyperparameters (multiplied by a prior, that can be uniform, and, essentially, a normalization factor). In order to construct this joint, global pdf we proceed hierarchically, in order to take into account all the different probability functions involved, starting by realizing that this pdf is equal to the product of all individual likelihood functions.
On the right-hand side, I show in detail how to build the joint pdf for a physical parameter a (note that a can represent more than one parameter). The method requires a model for the selection function of the survey and a noise model, as we are, in essence, deconvolving the intrinsic distribution from selection and photometric/spectroscopic-uncertainties effects. Once the joint pdf is built, it can be mapped and maximize, in order to constrain the hyperparameters of this intrinsic distribution.
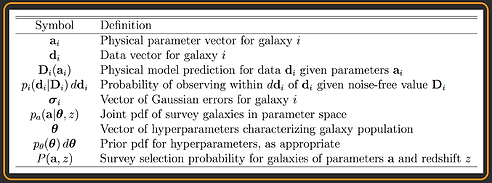
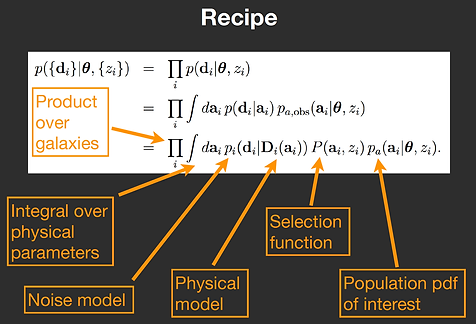
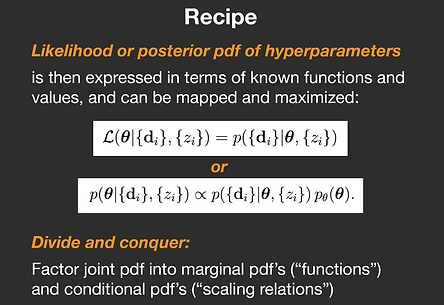
Stellar Population Synthesis
Stellar population synthesis models are tools for interpreting the integrated light (colors, line indices, and mass-to-light ratios) that we observe from the galaxies. Ideally, we want to determine what mix of stars and dust give rise to the observations. In their simplest form (the so-called single stellar populations or SSPs), they model the evolution of a coeval set of stars. More realistic models (composite stellar populations or CSPs) allow for star formation to occur during the lifes- pan of the galaxy (in a continuous way, in bursts or in a combination of both).
By comparing SPS models with observations, stellar population properties from galaxies can be derived. I have computed spectro-photometric stellar masses and other stellar population properties using the Flexible Stellar Population code (FSPS, Conroy et al. 2009). This project, called Granada FSPS, is an official SDSS-III galaxy product implemented in the BOSS pipeline and released for the first time as part of the SDSS DR10.
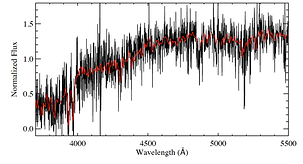
Example of BOSS spectrum (black) and a best-fit stellar population synthesis model (red), taken from the SDSS-III website(https://www.sdss3.org/dr9/algorithms/stellarpopulations.php#fitting)
Hubble Space Telescope
Astronomical observations from the ground suffer from degraded image quality due to turbulence in the Earth’s atmosphere, and to airglow at even the darkest of sites. The Hubble Space Telescope is a 2.4-m telescope that orbits at an altitude of 600 km above the Earth’s surface, and it is therefore free of these atmospheric drawbacks.
The sharpness and quality of images delivered by the Hubble is unmatched, and it allows us to make measurements that would otherwise be impossible.
I am currently partcipating in the recently-approved HST Cycle 23 project "Quantifying Cold Dark Matter Substructure with a Qualitatively New Gravitational Lens Sample" (P.I.: A. Bolton), which is aimed at measuring the subhalo mass function on scales that are hard to probe by different means.
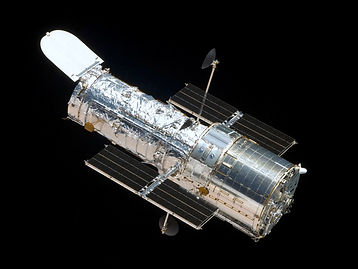